projects
TrAgS - DFG Individual Grant (2022-2025)
Tracking the use and adoption of agricultural technologies through satellite remote sensing and self-supervised deep learning
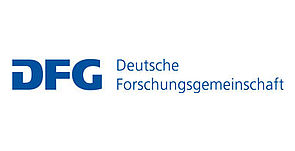
Background
Novel technologies and farm management systems are considered to be crucial to increase agriculture’s sustainability and resource use efficiency. However, our data sources that provide information on what technologies are actually being used on farms are very limited. This makes it difficult to assess if technologies can indeed realize their promised environmental effects and also limits our possibilities to study and understand technology diffusion processes.
Hypothesis and Aims
Satellite data and novel deep learning approaches offer currently unused opportunities that can help to overcome those data limitations. So far, however, those datasets are not fully exploited to study adoption behavior or diffusion processes. Further, although the existing data products offer substantial opportunities, they remain limited in terms of the regions/technologies they cover. Extending to other regions/technologies is limited because learning new machine learning models or extending existing models requires large amounts of labeled reference data (i.e., observations with known reference information) that are often lacking. The aims of this project are therefore twofold.
1) First, we aim to exploit data products derived from satellite data to study diffusion processes, particularly adoption (disadoption) dynamics and spatially mediated peer effects.
2) Secondly, we aim to significantly reduce the number of needed labeled reference data for deep learning approaches when extending the approach to other regions/technologies.
Approach
We exploit an existing large scale dataset for low tillage in the US derived from satellite data to study diffusion processes. Additionally, we intend to develop novel learning procedures, and we specifically explore if the inclusion of agronomic domain knowledge can help to reduce the required number of labeled reference data. We benchmark the developed approaches against existing US data and apply them to create new data products covering other regions (Germany) and technologies (low tillage and glyphosate usage). The derived dataset will be made available publicly. We will use it to derive diffusion dynamics for low tillage and glyphosate usage in Germany which can deliver an important contribution for the ongoing policy debate around those technologies.
Staff working on the project:
Principle Investigators:
- Hugo Storm (primarily working on aim 1)
- Ribana Roscher (primarily working on aim 2)
PhD Students:
- Not jet hired: Apply here
Interested in the project? Contact Hugo Storm (hugo.storm@ilr.uni-bonn.de)
DETECT - DFG Collaborative Research Centre 1502
Regional Climate Change: Disentangling the Role of Land Use and Water Management
The CRC1502 joins forces in the University of Bonn and its project partners to address one of the most challenging problems in our understanding of climate change.
While several continental regions on Earth are getting wetter, others are drying out not only in terms of precipitation but also measured by the increase or decrease in surface water, water stored in the soils, the plant root zone, and in groundwater. Observations, however, do not support a simple dry-gets-dryer and wet-gets-wetter logic and existing climate models fail to explain observed patterns of hydrological change sufficiently.
Project B04
Project B04 within the CRC seeks to create land use map with detailed crop cover information and uncertainty quantification. For this we aim to use Bayesian statistical approaches to merge a broad range of input data (existing land use maps, official statistics, sample data). The map will provide a consistent and complete time series for Europe with an unprecedented combination of spatial resolution and crop coverage. Furthermore, we aim to develop a methodology that allows combining data driven models, such as deep learning methods applied to satellite imagery, with econometric land-use models considering economic and biophysical information. For combining these currently distinct approaches, techniques from the field of probabilistic programming shall be explored. Both activities are targeted to improve our knowledge of past land use (changes), which is crucial to model future land use and the implications for regional climate change within the cluster.
Staff working on the project B04:
Principle Investigators:
PhD Students:
- Josef Baumer
- NaN
Mind-Step (2019-2023)
Mind-Step, Modelling Individual Decisions to Support the European Policies Related to Agriculture
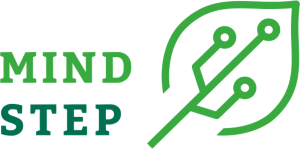
Overview:
The 4-year Horizon 2020 project MIND-STEP (770747, 2019-2023) - Modelling Individual Decisions to Support the European Policies Related to Agriculture, funded by the EU, aims to improve exploitation of available agricultural and biophysical data and will include the individual decision making (IDM) unit in policy models.
Objectives of the project:
To develop a highly modular and customizable suite of Individual Decision Making (IDM) models focusing on behaviour of individual agents in the agricultural sector to better analyze impacts of policies To develop linkages between the new IDM models and current models used at the European Commission to improve the consistency and to broaden the scope of the analysis of policies To develop an integrated data framework to support analysis and monitoring of policies related to agriculture To apply the MIND STEP model toolbox to analyze regional and national policies and selected EU CAP reform options and global events affecting the IDM farming unit, working together with policymakers, farmers and other stakeholders To safeguard the governance and future exploitation of the MIND STEP model toolbox
Partners:
John Helming, WUR, The Netherlands (coordinator) 11 partners from seven countries in Europe (The Netherlands, Germany, Austria, Italy, France, Spain, Norway and Hungary)
Group Contribution
- Hugo Storm Work Package leader (WP4), Task Leader T4.5
More information on the MIND-STEP project can be found at its home page. mind-step.eu/partners/ubo
PhenoRob - DFG Cluster of Excellence
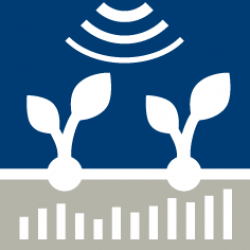
PhenoRob, Robotics and Phenotyping for Sustainable Crop Production